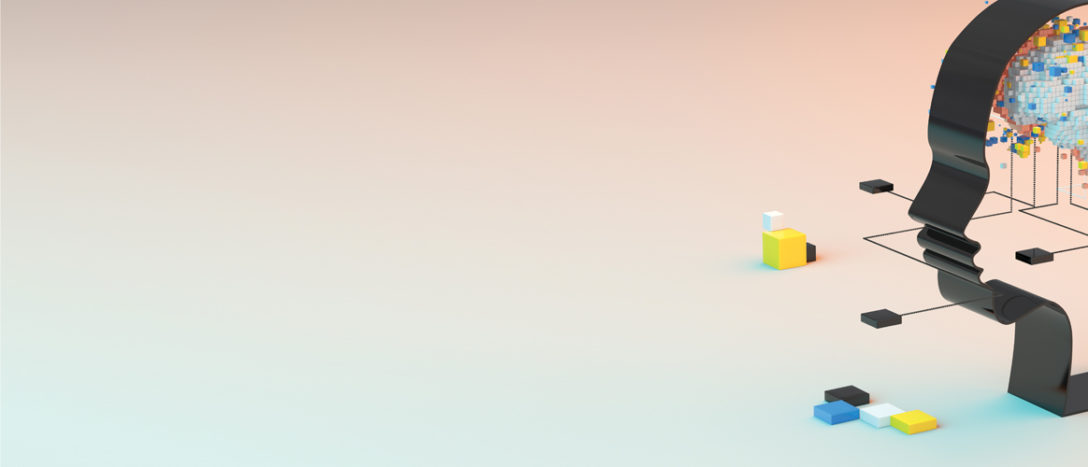
AI: Embracing the digital frontier
Infrastructure asset managers are finally welcoming the operational benefits of holistic digitalisation.
A verification email is on its way to you. Please check your spam or junk folder just in case.
If you do not receive this within five minutes, please try to sign in again. If the problem persists, please email: subscriptions@pei.group .Issues with signing in? Click here
Don't have an account? Register now
Infrastructure asset managers are finally welcoming the operational benefits of holistic digitalisation.
Nearly there!
A verification email is on its way to you. Please check your spam or junk folder just in case.
Copyright PEI Media
Not for publication, email or dissemination